Q&A with HOCINE OUSMER
- Trusted Magazine
- Feb 11, 2023
- 3 min read
Updated: Jul 21, 2023
Exclusive Trusted Magazine Q&A with Hocine Ousmer, Head Digital & Communication Manager@Datategy

Can you tell us a bit about yourself and how you came up with the TPES Transparency Privacy Explainability Score formula?
I am Hocine OUSMER, passionate about digital and new technologies. I have spent most of my career popularising the understanding of and access to new technologies among my different collaborators.
Among the topics that I am currently passionate about and that fascinates many people is artificial intelligence, sometimes praised and sometimes criticized, it does not leave indifferent.
Proponents point to the benefits of its use and detractors point to the threats that misuse can generate. The subject of transparency and privacy is coming up more and more often, and that's why I thought of an AI evaluation barometer on this subject.
What does the Transparency Privacy Explainability Score (TPES) mean?
The transparency Privacy Explainability Score (TPES) is a metric that evaluates the level of transparency, privacy, and explainability in a machine learning model or AI system. The score is used to assess the level of trust and accountability in the AI system, and to identify areas for improvement in terms of transparency, privacy, and explainability. A high TPES score indicates that the AI system is open, transparent, and easily understandable, while a low TPES score may indicate the need for improvements in terms of privacy and explainability.
How is TPES calculated?
Transparency Privacy Explainability Score is a formula for evaluating artificial intelligence platforms in three areas:
1 Transparency:
- Transparency of data sources and preparation methods.
- Access and sharing by third parties.
2 Privacy:
- Policies on data collection and storage use.
- Compliance with privacy laws and regulations (GDPR, CCPA..).
3 Explainability :
- Access to the model architecture and training data.
- Explanation of the AI decision-making process
(ability to understand the impact of individual input variables on the output).
A score out of five is given to each category with a final score out of ten.
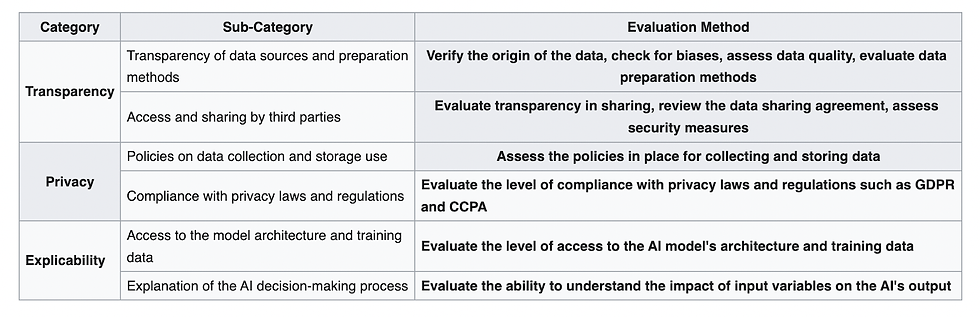
Can you give us an example of how TPES could be used in real-world applications?
Sure! For example, in the healthcare industry, an AI model could be used to diagnose diseases based on medical images. The TPES score could be used to determine the transparency, privacy, and explainability of the model and help healthcare providers determine whether it can be trusted for clinical decision-making. Similarly, in the financial industry, the TPES score could be used to evaluate the transparency and reliability of AI models used for investment and risk management.
How does TPES differ from other explainability scores and metrics currently in use?
TPES is unique in that it takes into account not just the explainability of an AI model but also its transparency and privacy. Other explainability scores may only evaluate a model's ability to provide explanations for its predictions, while TPES takes a more comprehensive approach by considering all three key factors. Additionally, TPES is designed to be flexible and adaptable, so it can be applied to a wide range of AI models and use cases.
What do you see as the future of artificial intelligence and how do you see TPES evolving with it?
I see a bright future for AI, with continued advancements in areas such as robotics, autonomous systems, and personalized medicine. At the same time, I also see a need for increased attention to be paid to the ethical and societal implications of AI, such as privacy, transparency, and accountability.
In terms of TPES, I see it evolving alongside the development of AI. As AI continues to advance, TPES will need to adapt and incorporate new metrics and algorithms that are better suited for assessing the latest AI models. Additionally, I believe that TPES will become increasingly important as organizations and individuals seek ways to assess the transparency, privacy, and explainability of AI models in a rigorous and objective manner.
What challenges do you see in the development and deployment of AI, and how can TPES help to address these challenges?
One of the biggest challenges in the development and deployment of AI is ensuring that it is trustworthy and trustworthy. This means ensuring that AI models are transparent in their decision-making processes, that they respect the privacy of individuals and organizations, and that they can be easily explained to those who need to understand how they work.
Another challenge is ensuring that AI models are fair and unbiased. For example, AI models used in hiring and lending decisions need to be free of gender and racial biases. TPES can help address these challenges by providing a comprehensive and objective measure of an AI model's performance in terms of transparency, privacy, and explainability. By using TPES, organizations and individuals can make informed decisions about the use of AI and ensure that they are using models that are trustworthy, fair, and responsible.
Finally , I invite the entire data science and artificial intelligence community to contribute to the further development of this new approach.
Commentaires